– Europe/Lisbon
Room P3.10, Mathematics Building — Online
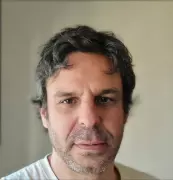
Luís Carvalho, CAMGSD & ISCTE
Aspects of approximation, optimization, and generalization in Machine Learning I
This talk offers a leisurely-paced and informal introduction to some classical results at the intersection of mathematics and machine learning theory. We will explore the subject through three central lenses: approximation, optimization, and generalization. Particular attention will be given to universal approximation theorems, which illustrate the expressive power of neural networks. The focus is on foundational ideas and mathematical intuition, I will also highlight some limitations of these classical tools. The goal is not to be exhaustive, but to offer a broad perspective and present a few selected proofs related to expressivity along the way.
References
- A. Pinkus. Approximation theory of the MLP model in neural networks. Acta Numerica, 1999.
- J. Berner, P. Grohs, G. Kutyniok and P. Petersen. The Modern Mathematics of Deep Learning, in: Mathematical Aspects of Deep Learning, CUP, 2023.