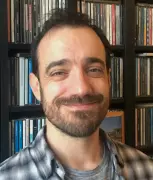
13:00– New schedule
Room P3.10, Mathematics Building
João Costa, CAMGSD & ISCTE
Introduction to Deep Learning for mathematicians (I)